A climate projection is the simulated response of the climate system under a scenario of future greenhouse gas (GHG) emissions or concentrations, usually derived from global climate models (GCM). A GCM is a representation of the climate system based on the physical, chemical and biological properties of its components, their interactions and feedback processes. Climate projections depend on the emissions scenario used, which in turn is based on assumptions regarding future socioeconomic and technological developments. GCM results have a resolution of approximately 100 or 200 km, which is not practical for assessing agricultural landscapes. Therefore, we use downscaled climate projections, interpolated at 5x5 km, which allows users to interpret the data at the district/municipality level. This approach relies on two key assumptions: first, that climatic changes vary only over large distances, and second, that the relationship between variables in the baseline remains valid in the future.
As for any future outlook, the models we use have a considerable degree of uncertainty and should be considered as projections of possible futures, not predictions. Climate projections are usually made by combining several independent models, to create a so-called ‘consensus’ model. GCMs currently available show a high level of agreement on an increase of temperature, but disagreement about the regional and seasonal distribution of precipitation (rainfall). The resulting consensus model of the independent projections is therefore, to a large degree, influenced by the temperature increase, while precipitation disagreement is masked (i.e., it can only be reflected to a certain extent in the final outcome). In our case, for example, we use floating bars to show the values ranges of different projections when show-casing future precipitation values.
Also, at least two major tendencies are important to bear in mind when discussing the impacts of climate change: change in mean values and change in frequency of extreme events (variability). A change in mean values shifts what is experienced as ‘normal’, an ‘extreme event’ or the ‘new extreme’. For example: for a given location formerly accustomed to a monthly mean temperature of 27°C, 30°C may become the new ‘normal’ temperature in summer, and what used to be considered extreme at 37°C is now surpassed frequently so that the ‘new extreme’ is found at 45°C, and so forth. When looking at the frequency of extreme events we need to look at climate variability. A change in frequency can mean, for example, that the same amount of monthly rainfall falls, but that it is now more often concentrated into two days instead of being spread throughout the month, leading to an increase in flooding and water erosion. For the combined change of means and variability see also Figure 1.
Our tool focusses on changes in means (i.e., ‘what is normal’). Mean changes in temperature (minimum, mean and maximum) and rainfall (monthly/yearly) can be modelled and displayed in quite a straightforward way and are included in our platform. However, changes in variability are not included yet, except for some of the climate hazard indicators that make specific reference to it.
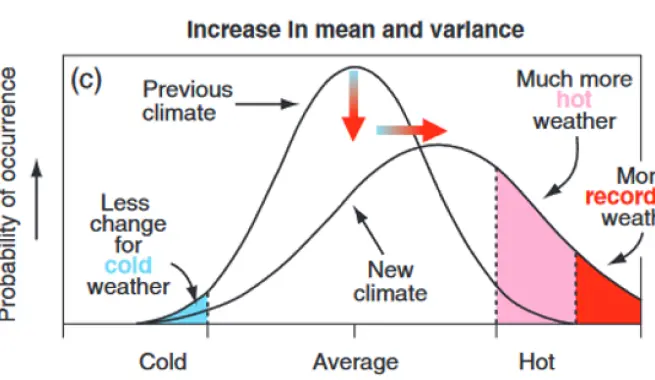
Source: Houghton JT et al., eds. (2001). Climate change 2001: the scientific basis. Contribution of Working Group I to the Third Assessment Report of the Intergovernmental Panel on Climate Change. Cambridge, Cambridge University Press. (Fig 2.32, to be found on p. 155). source link.